This course provides an overview of some different concepts underpinning Generative AI, their mathematical principles, and their applications in engineering. The focus will be on the practical implementation of generative AI including, neural networks, attention mechanism, and advanced deep learning models.

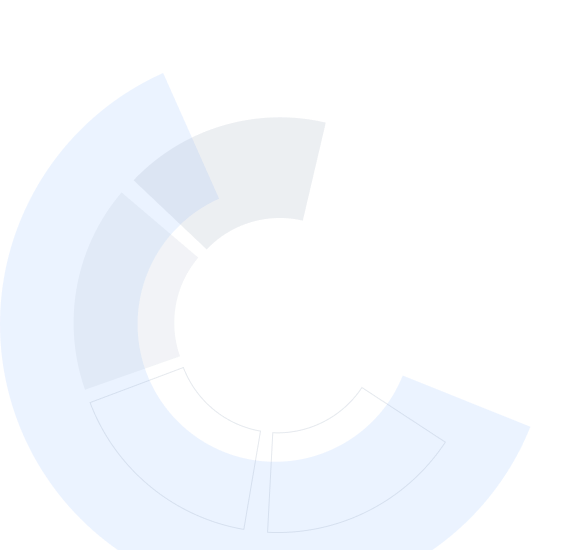
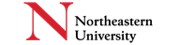
Détails à connaître

Ajouter à votre profil LinkedIn
avril 2025
9 devoirs
Découvrez comment les employés des entreprises prestigieuses maîtrisent des compétences recherchées
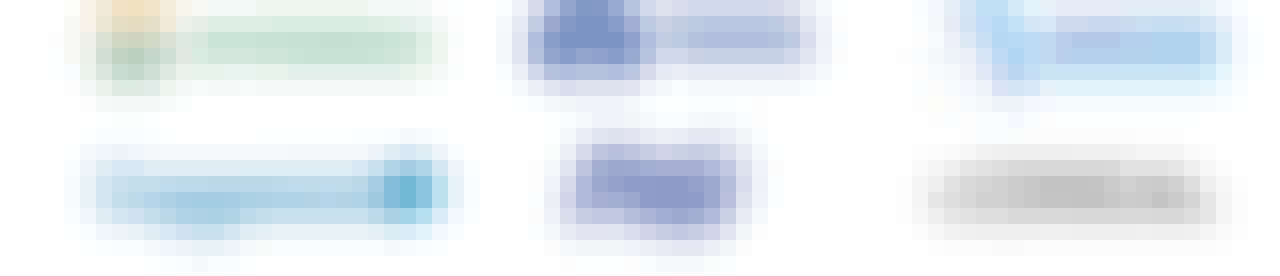
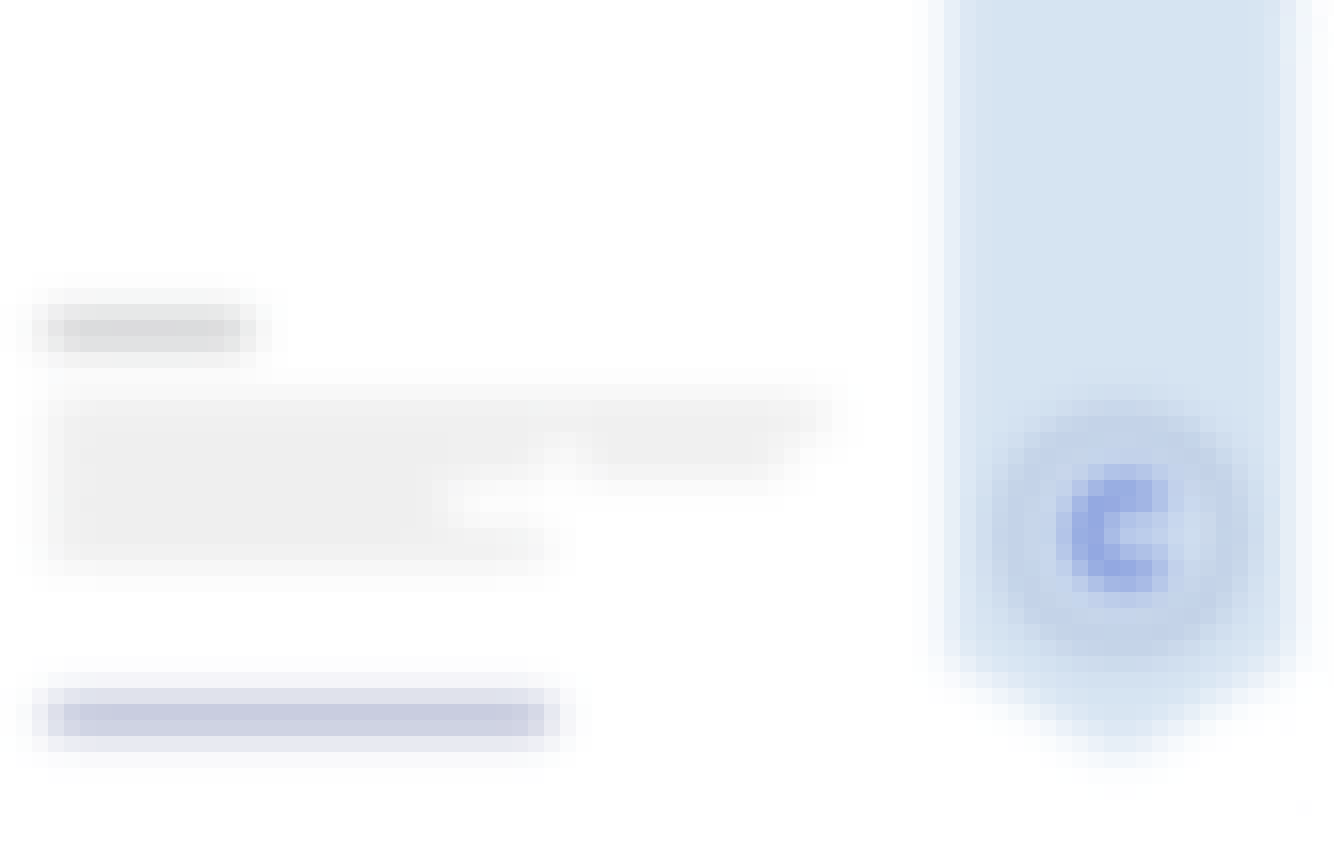
Obtenez un certificat professionnel
Ajoutez cette qualification à votre profil LinkedIn ou à votre CV
Partagez-le sur les réseaux sociaux et dans votre évaluation de performance
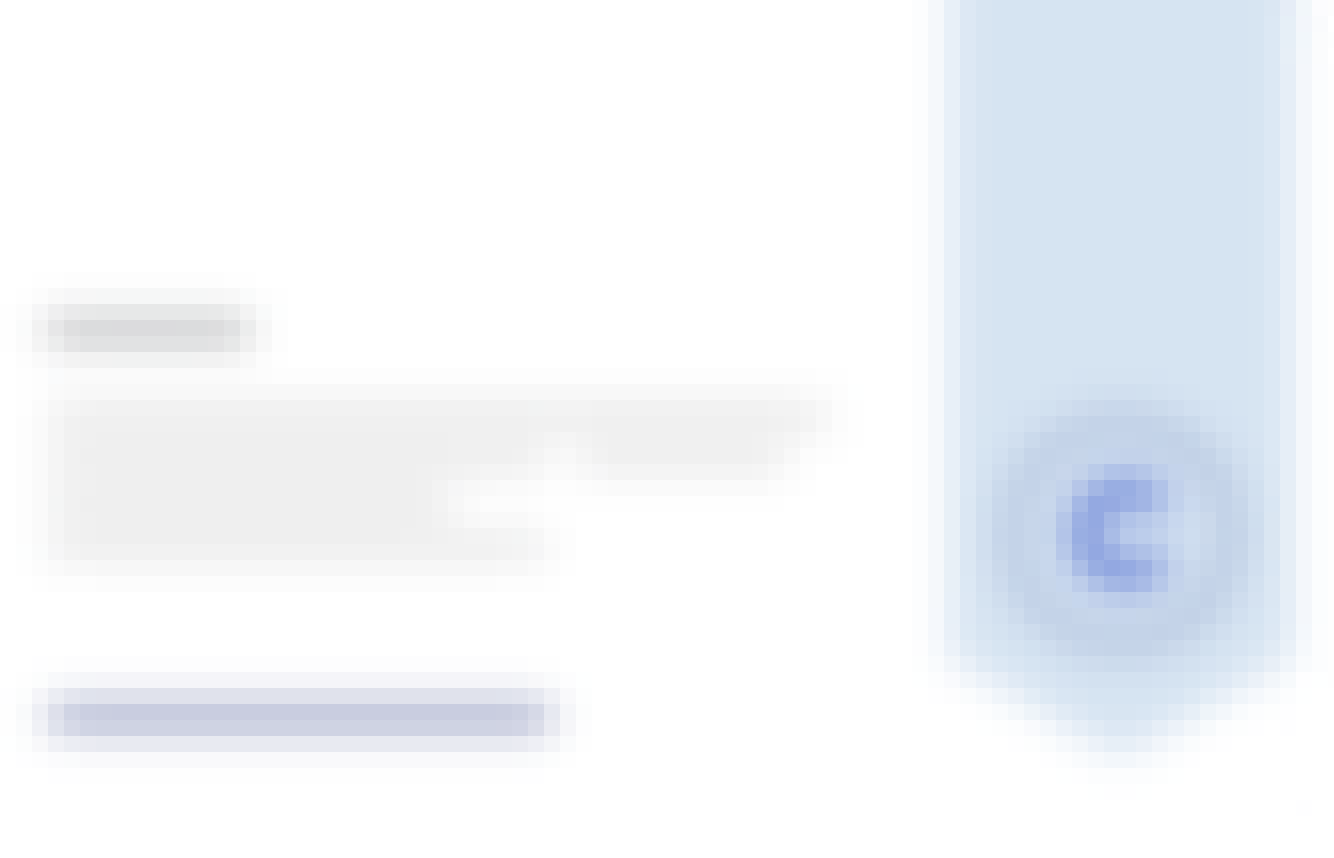
Il y a 4 modules dans ce cours
In this module, you will explore the foundations of neural networks, including perceptrons, architectures, and learning algorithms. You will dive deeply into optimization methods critical for efficient training, focusing on advanced techniques like Newton’s and quasi-Newton methods, momentum, RMSProp, and Adam optimization algorithms.
Inclus
6 vidéos15 lectures2 devoirs2 sujets de discussion
This module guides you through the mathematical approaches to regularization techniques that enhance neural network generalization and prevent overfitting. You will analyze concepts including Stein’s unbiased risk estimator, eigen decomposition, ensemble methods, dropout mechanisms, and advanced normalization techniques such as batch normalization.
Inclus
4 vidéos17 lectures2 devoirs1 sujet de discussion
In this module, you will examine convolutional neural networks (CNNs), including convolution operations, parameter sharing, kernel methods, and multi-dimensional data structures. You'll explore advanced CNN architectures, regularization, normalization techniques, and the implications of random kernels on network learning behavior.
Inclus
5 vidéos31 lectures2 devoirs1 sujet de discussion
In this module, you will analyze the maths underpinning generative models and maximum likelihood estimation (MLE). You will explore divergence metrics such as Kullback-Leibler divergence, Bayesian network structures, and autoregressive modeling methods, focusing on their theoretical foundations and practical implications.
Inclus
6 vidéos33 lectures3 devoirs1 sujet de discussion
Instructeur

Offert par
Recommandé si vous êtes intéressé(e) par Machine Learning
University of Colorado Boulder
Duke University
Google Cloud
Pour quelles raisons les étudiants sur Coursera nous choisissent-ils pour leur carrière ?





Ouvrez de nouvelles portes avec Coursera Plus
Accès illimité à 10,000+ cours de niveau international, projets pratiques et programmes de certification prêts à l'emploi - tous inclus dans votre abonnement.
Faites progresser votre carrière avec un diplôme en ligne
Obtenez un diplôme auprès d’universités de renommée mondiale - 100 % en ligne
Rejoignez plus de 3 400 entreprises mondiales qui ont choisi Coursera pour les affaires
Améliorez les compétences de vos employés pour exceller dans l’économie numérique
Foire Aux Questions
Access to lectures and assignments depends on your type of enrollment. If you take a course in audit mode, you will be able to see most course materials for free. To access graded assignments and to earn a Certificate, you will need to purchase the Certificate experience, during or after your audit. If you don't see the audit option:
The course may not offer an audit option. You can try a Free Trial instead, or apply for Financial Aid.
The course may offer 'Full Course, No Certificate' instead. This option lets you see all course materials, submit required assessments, and get a final grade. This also means that you will not be able to purchase a Certificate experience.
When you purchase a Certificate you get access to all course materials, including graded assignments. Upon completing the course, your electronic Certificate will be added to your Accomplishments page - from there, you can print your Certificate or add it to your LinkedIn profile. If you only want to read and view the course content, you can audit the course for free.
You will be eligible for a full refund until two weeks after your payment date, or (for courses that have just launched) until two weeks after the first session of the course begins, whichever is later. You cannot receive a refund once you’ve earned a Course Certificate, even if you complete the course within the two-week refund period. See our full refund policy.
Plus de questions
Aide financière disponible,